Debezium Blog
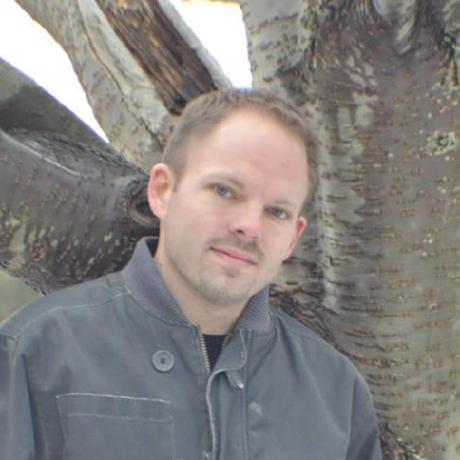
While it has only been two short weeks since our first preview release for the Debezium 2.5 release stream, I am happy to announce the immediate availability of the next preview release, Debezium 2.5.0.Alpha2.
This release includes a variety of improvements, batch support for the JDBC Sink connector, seamless support for MongoDB documents that exceed the 16MB barrier, MySQL 8.2 compatibility, and signal improvements for SQL Server. Additionally, this release includes a variety of bug fixes and several breaking changes.
Let’s take a closer look at these changes and improvements that are included in Debezium 2.5.0.Alpha2; as always, you can find the complete list of changes for this release in the release notes. Please remember to take special note to any breaking changes that could affect your upgrade path.
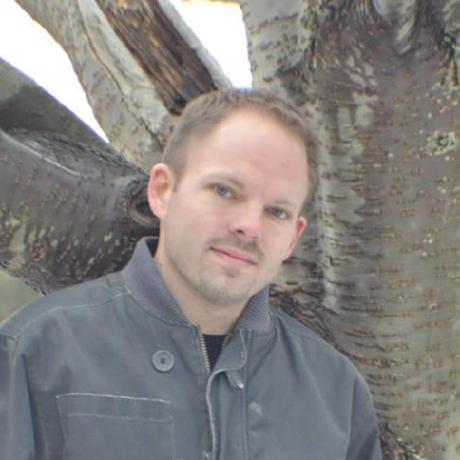
It’s been about three weeks since we released Debezium 2.4, and in that time the team has been diligently working on what comes next in the evolution of Debezium. I am pleased to announce that today we have released Debezium 2.5.0.Alpha1, the first preview release of Debezium’s 2.5 release stream. This release includes many new exciting features as well as bug fixes, e.g. a brand-new IBM Informix connector, a preview support for MariaDB with the...
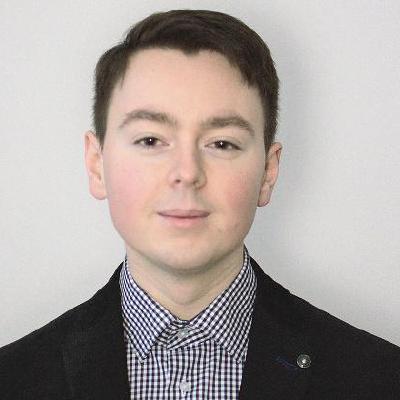
With Debezium 2.3, we introduced a preview of a brand new Debezium Operator with the aim to provide seamless deployment of Debezium Server to Kubernetes (k8s) clusters. The Debezium 2.4.0.Final release brings the next step towards the full support of this component. With this release, we are happy to announce that Debezium Operator is now available in the OperatorHub catalog for Kubernetes as well as in the community operator catalog embedded in the OpenShift and OKD distributions. The operator remains in the incubation phase; however, the full support of this component is approaching fast.
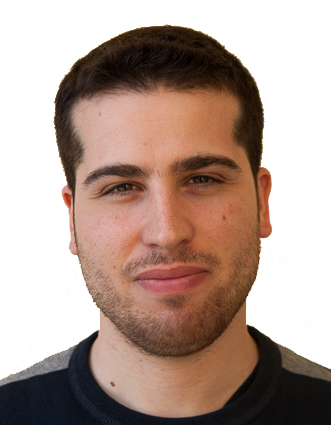
Welcome to the third installment of our series on Debezium Signaling and Notifications. In this article, we continue our exploration of Debezium signaling and notifications. In particular, we will delve into how to enable and manage these features using the JMX channel.
We will also explore how to send signals and get notifications through the REST API leveraging Jolokia.
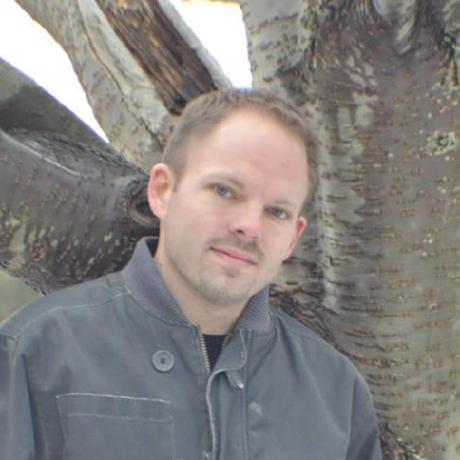
As the summer months wind down and we enter autumn with cooler temperatures, the team has diligently prepared the next major milestone of Debezium. It’s my pleasure to announce the immediate release of the next minor version, Debezium 2.4.0.Final.
As the team begins the journey toward the next development iteration, let’s take a moment and review all the new features, changes, and improvements that are included in Debezium 2.4, which includes 231 issues resolved by 68 unique contributors.