Debezium Blog
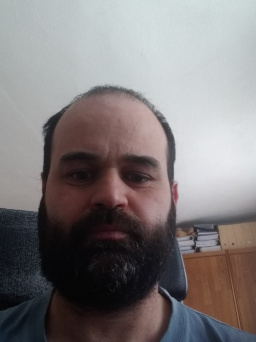
Debezium provides a way to run the connectors directly within Debezium from the very beginning of the project. The way how it was provided has changed over the time and it still evolves. This article will describe another evolution step in this regard - new implementation of Debezium engine.
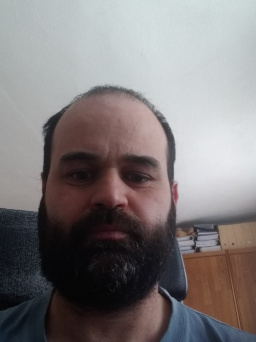
In the previous blog post, we have shown how to leverage Debezium to train neural-network model with the existing data from the database and use this pre-trained model to classify images newly stored into the database. In this blog post, we will move it one step further - we will use Debezium to create multiple data streams from the database and use one of the streams for continuous learning and to improve our model, and the second one for making predictions on the data. When the model is constantly improved or adjusted to recent data samples, this approach is known as online machine learning. Online learning is only suitable for some use cases, and implementing an online variant of a given algorithm may be challenging or even impossible. However, in situations where online learning is possible, it becomes a very powerful tool as it allows one to react to the changes in the data in real-time and avoids the need to re-train and re-deploy new models, thus saving the hardware and operational costs. As the streams of data become more and more common, e.g. with the advent of IoT, we can expect online learning to become more and more popular. It’s usually a perfect fit for analyzing streaming data in use cases where it’s possible.
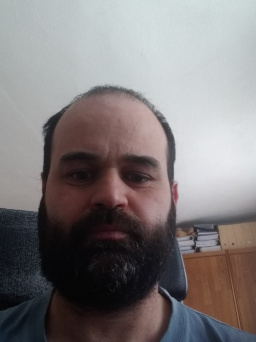
Every now and then there is a questions in the Debezium chat or on the mailing list how to ensure exactly-once delivery of the records produced by Debezium. So far Debezium aimed only for at-least-once delivery. This means Debezium guarantees every single change will be delivered and there is no missing or skipped change event. However, in case of failures, restarts or DB connection drops, the same event can be delivered more than once. Typical scenario is that the event is delivered twice - once before failure/restart and second time after that. Exactly-once delivery (or semantic) provides stronger guarantee - every single message will be delivered and at the same time there won’t be any duplicates, every single message will be delivered exactly once. So far our answer was that the users have to implement their own deduplication system if they need exactly-once delivery. However, with Kafka Connect support for exactly-once delivery, it seems we can provide exactly-once delivery for Debezium connectors out-of-the-box, only with a little configuration change.
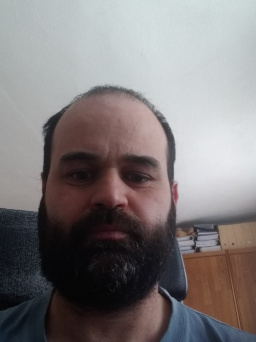
With the recent success of ChatGPT, we can observe another wave of interest in the AI field and machine learning in general. The previous wave of interest in this field was, at least to a certain extent, caused by the fact that excellent ML frameworks like TensorFlow, PyTorch or general data processing frameworks like Spark became available and made the writing of ML models much more straightforward. Since that time, these frameworks have matured, and writing models are even more accessible, as you will see later in this blog. However, data set preparation and gathering data from various sources can sometimes take time and effort. Creating a complete pipeline that would pull existing or newly created data, adjust it, and ingest it into selected ML libraries can be challenging. Let’s investigate if Debezium can help with this task and explore how we can leverage Debezium’s capabilities to make it easier.
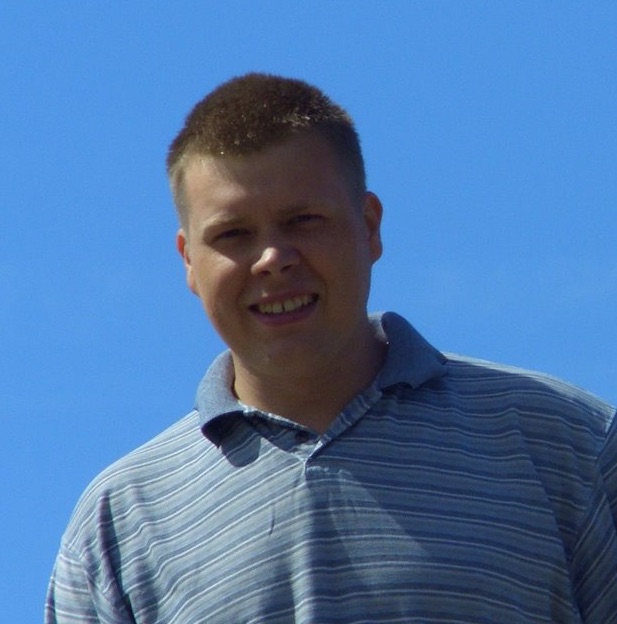
As a follow up to the recent Building Audit Logs with Change Data Capture and Stream Processing blog post, we’d like to extend the example with admin features to make it possible to capture and fix any missing transactional data.
In the above mentioned blog post, there is a log enricher service used to combine data inserted or updated in the Vegetable database table with transaction context data such as
-
Transaction id
-
User name who performed the work
-
Use case that was behind the actual change e.g. "CREATE VEGETABLE"
This all works well as long as all the changes are done via the vegetable service. But is this always the case?
What about maintenance activities or migration scripts executed directly on the database level? There are still a lot of such activities going on, either on purpose or because that is our old habits we are trying to change…
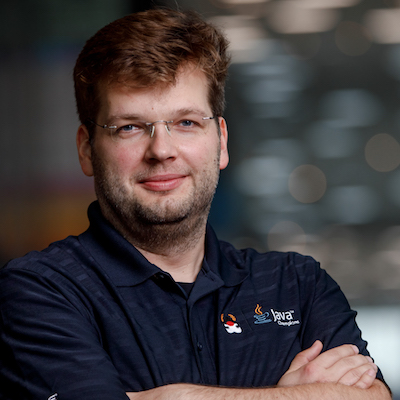
It is a common requirement for business applications to maintain some form of audit log, i.e. a persistent trail of all the changes to the application’s data. If you squint a bit, a Kafka topic with Debezium data change events is quite similar to that: sourced from database transaction logs, it describes all the changes to the records of an application. What’s missing though is some metadata: why, when and by whom was the data changed? In this post we’re going to explore how that metadata can be provided and exposed via change data capture (CDC), and how stream processing can be used to enrich the actual data change events with such metadata.
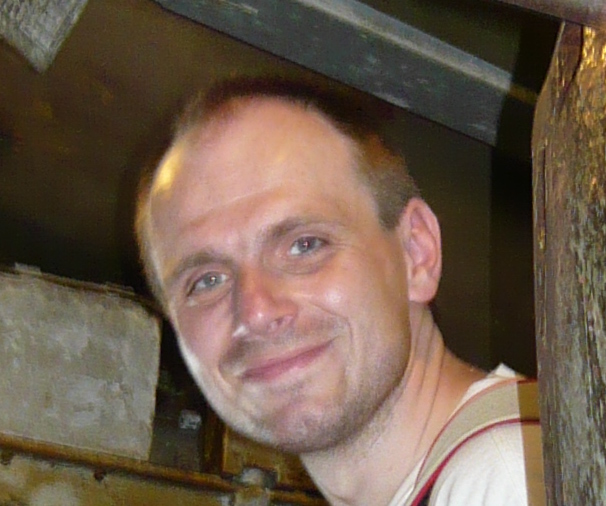
Last week’s announcement of Quarkus sparked a great amount of interest in the Java community: crafted from the best of breed Java libraries and standards, it allows to build Kubernetes-native applications based on GraalVM & OpenJDK HotSpot. In this blog post we are going to demonstrate how a Quarkus-based microservice can consume Debezium’s data change events via Apache Kafka. For that purpose, we’ll see what it takes to convert the shipment microservice from our recent post about the outbox pattern into Quarkus-based service.
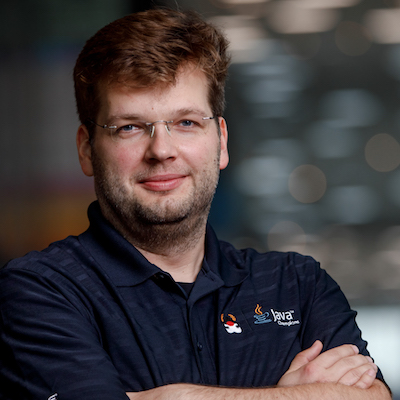
As part of their business logic, microservices often do not only have to update their own local data store, but they also need to notify other services about data changes that happened. The outbox pattern describes an approach for letting services execute these two tasks in a safe and consistent manner; it provides source services with instant "read your own writes" semantics, while offering reliable, eventually consistent data exchange across service boundaries.