Debezium Blog
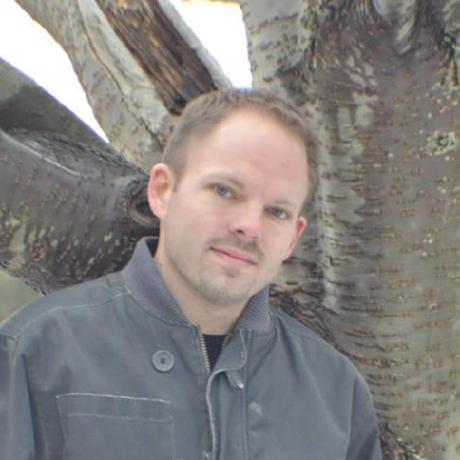
We are happy to announce the first pre-release of Debezium 3, 3.0.0.Alpha1. This release, albeit smaller than our normal pre-releases, is highly focused on a few key points, such as testing the release process with Java 17/21; however, it also includes several new features. Let’s take a moment and talk about the upcoming breaking changes in-depth and the new features you will find.
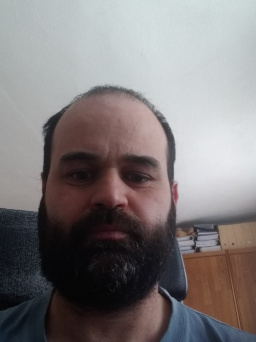
Debezium provides a way to run the connectors directly within Debezium from the very beginning of the project. The way how it was provided has changed over the time and it still evolves. This article will describe another evolution step in this regard - new implementation of Debezium engine.
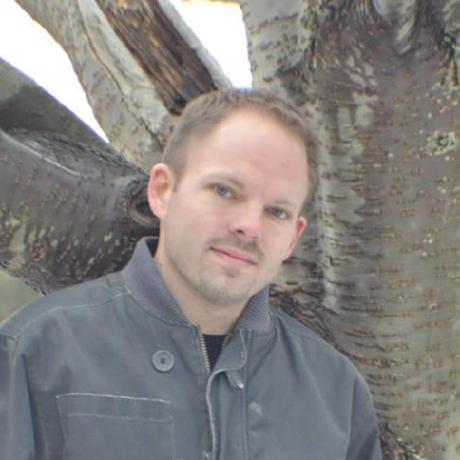
As the team leaps into Q3, we’re happy to announce the fruits of our Q2 work, Debezium 2.7.0.Final is now generally available. This release includes changes for 140 issues with contributions from over 51 contributors. Let’s take a moment and review all the changes.
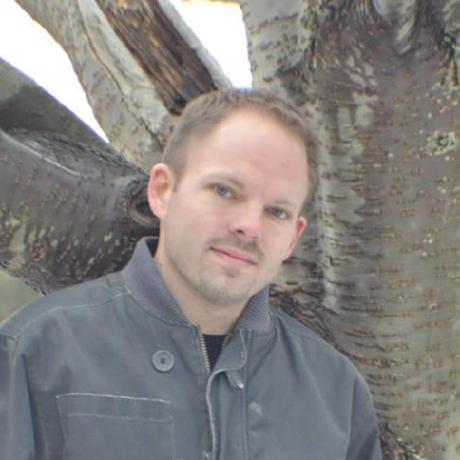
Although half of 2024 is nearly behind us, the team is pleased to announce the first beta preview release for Debezium, 2.7.0.Beta1.
This release includes incubating support for Db2 on z/OS, authentication and encryption with NATS JetStream, improvements for the MariaDB JDBC sink dialect, JMX Exporter with Debezium Server images, configurable metrics in Debezium Operator, and more.
Let’s walk through all the highlights and discuss these in more depth…
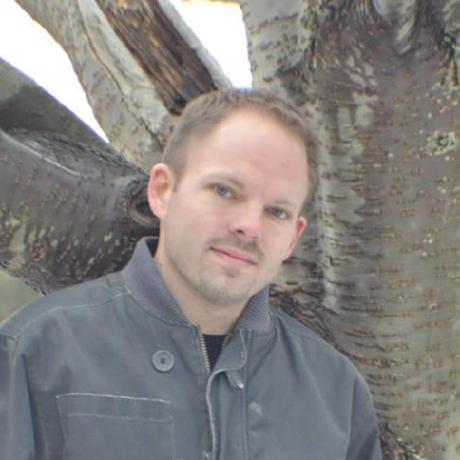
I’m pleased to announce the immediate availability of Debezium 2.6.2.Final. This release is the second maintenance release that focuses on addressing several critical stability issues with the 2.6.1.Final release, support for Oracle database query filtering with more than one thousand tables, fixed race condition with PostgreSQL offset flushing, fixed Avro compatibility, and more.
Let’s take a few moments and dive into these and more…