Debezium Blog
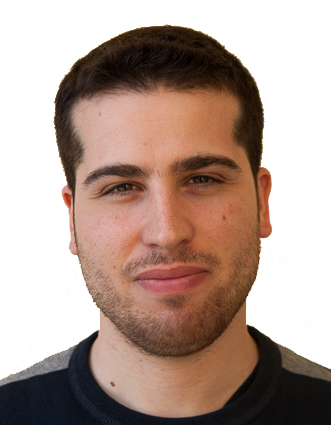
A year ago we began this incredible journey to create a modern approach to Change Data Capture. We had the desire to create a tool where you can focus on your data, defining how it flows from sources to destinations, with a pipeline-based approach. All this paired with a new and modern user interface to simplify interaction with it.
We named it Debezium Management Platform, or if you prefer, just Debezium Platform.
We are excited that Debezium 3.1 is the first official release of this years-long effort.
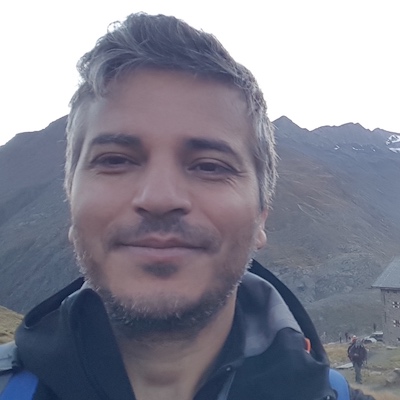
When it comes to replicating operational data for analytics, Change Data Capture (CDC) is the gold standard. It offers scalability, near real-time performance, and captures all data modifications, ensuring your analytical datasets are always up-to-date. Debezium is a leading tool in this space, connecting to a wide range of databases and exporting CDC events in various formats like JSON and Avro, making integration with diverse systems a breeze.
While Debezium itself is a Java-based project, the data engineering world increasingly relies on Python. This blog post demonstrates how to leverage Debezium within a Python environment, using pydbzengine. We’ll explore how to use these technologies to build a robust and scalable CDC solution.
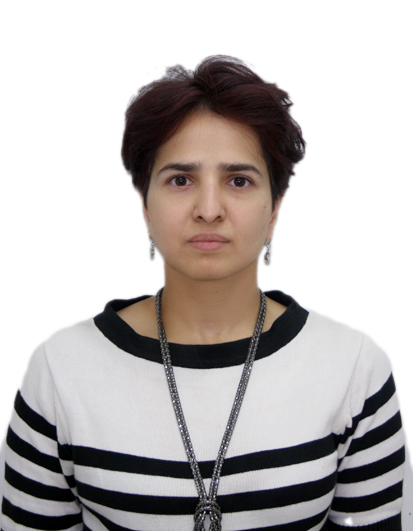
In this post, we are going to talk about a CDC-CQRS pipeline between a normalized relational database, MySQL, as the command database and a de-normalized NoSQL database, MongoDB, as the query database resulting in the creation of DDD Aggregates via Debezium & Kafka-Streams.